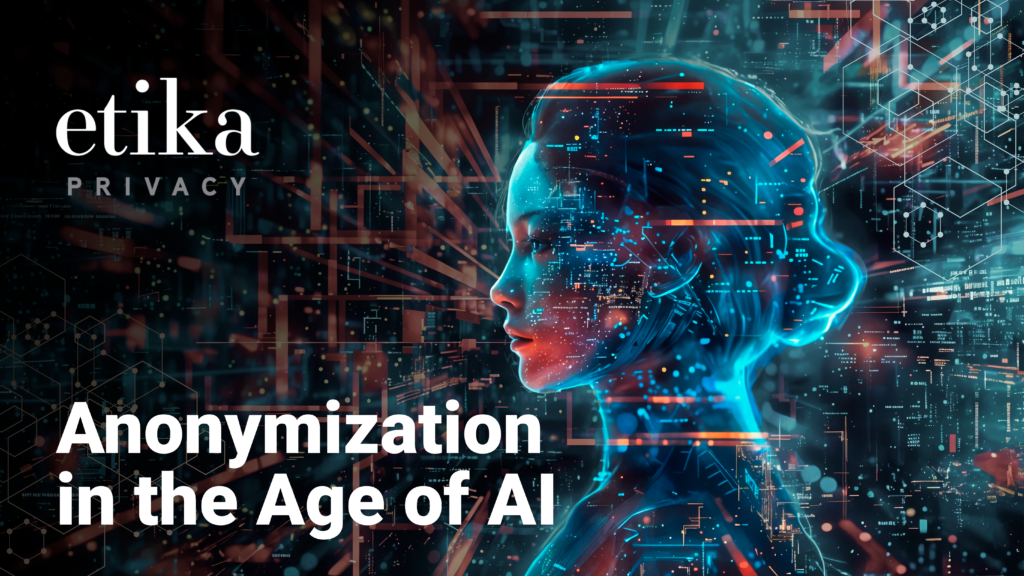
Personal information is defined, under PIPEDA Section 2(1), as “information about an identifiable individual.” Similarly, Québec’s Law 25 defines personal information as “any information which relates to a natural person and directly or indirectly allows that person to be identified.” Section 23 of Québec’s Law 25 defines anonymized personal information as “information concerning a natural person […]if it is, at all times, reasonably foreseeable in the circumstances that it irreversibly no longer allows the person to be identified directly or indirectly.”
Hence, when anonymization is done effectively, the data is no longer considered personal information and therefor Law 25 no longer applies.
Achieving anonymization can be challenging, depending on the data set’s characteristics, e.g., its size, content, and format, or even impossible. That may be the case when the intended use of the data can only be achieved by retaining personal identifiers to such an extent that identification will likely be possible. Another factor is that further advances in technology may lead to the re-identifiability of data that was previously considered anonymized.
This article focuses on the requirements for data anonymization in Canada, specifically under the currently strictest law and its accompanying regulation, Québec’s Law 25. We then look at compliance challenges in the context of AI, specifically LLMs, and existing guidance and proposed solutions. Lastly, we introduce a technological solution that can support data anonymization efforts, Private AI’s de-identification software.
Anonymization Requirements in Canada
Canada’s federal privacy law that is currently in effect, PIPEDA, is mostly silent on what is required to achieve anonymization. It only mentions the concept once as a legitimate alternative to disposing of data, without so much as defining anonymization. The proposed Bill C-27, including the proposed Consumer Privacy Protection Act (CPPA), which aims at modernizing PIPEDA, defines anonymization and distinguishes between anonymization and de-identification. According to the proposed CPPA, anonymized personal information means “to irreversibly and permanently modify personal information, in accordance with generally accepted best practices, to ensure that no individual can be identified from the information, whether directly or indirectly, by any means”. Although strict, this definition leaves room for interpretation when referring to “generally accepted best practices”. It is currently also questionable whether the Bill will become law before the next Canadian election, after which its fate remains uncertain.
Much more detailed guidance exists under Québec’s Law 25, and more precisely under its latest anonymization regulation, which came into force in May 2024.
The anonymization process required under the Regulation is as follows:
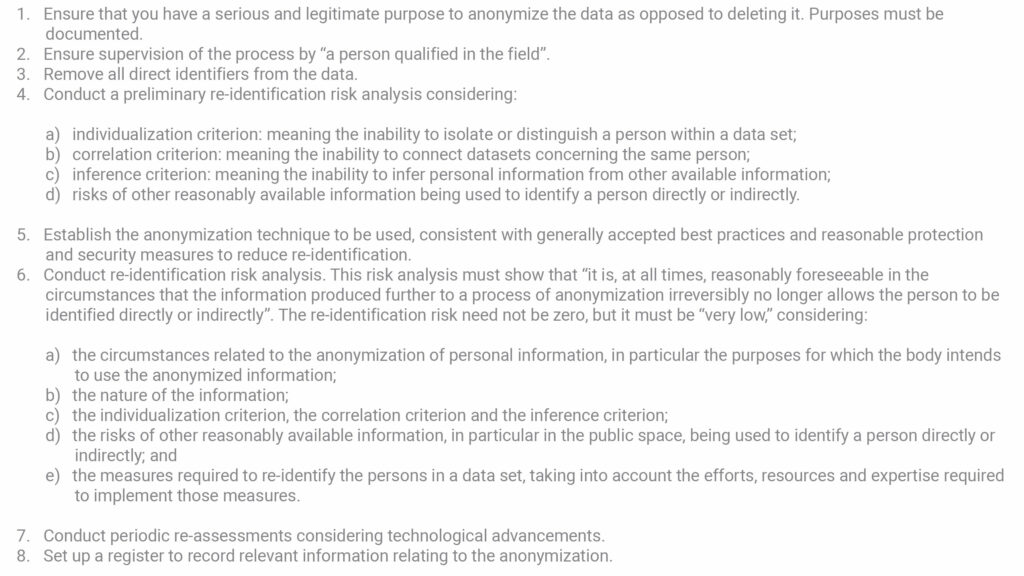
Consequences for Contravention of the Anonymization Provision
Before we dive into the compliance challenges in the context of AI, we briefly look at the possible consequences for failing to anonymize the data in accordance with the Regulation, so we know what might be at stake.
There exist significant sanctions under Law 25, namely administrative monetary penalties that have less the purpose to punish rather than to encourage compliance, and penalties including criminal offences punishable by fines.
Not properly anonymizing personal data could attract consequences under both regimes. We’re setting out the exact provisions below, but in summary, the maximum fine for businesses failing to properly anonymize data is $25 million or, if greater, the amount corresponding to 4% of worldwide turnover for the preceding fiscal year. Note that there is, at the time of writing, no precedent of an enforcement action that has been taken for such a violation. We can make the educated guess, however, that it would have to be an egregious one to attract such a large fine.
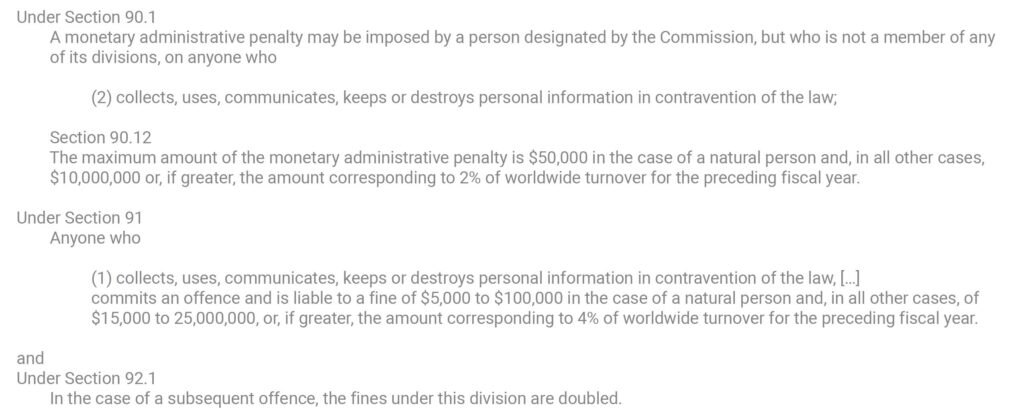
Compliance Challenges in the AI Context
Data anonymization is a great data protection technique including in the AI context because it minimizes risks to your organization. In addition, as we mentioned, it means that privacy laws do not apply, lowering the compliance burden.
However, it presupposes that the organization’s use case allows for anonymization. If anonymization can’t be achieved without overly impacting the accuracy of results or the usefulness of the AI system in general, that is not in itself a problem; it means, though, that various privacy requirements must be met with regards to the data.
But while there is no explicit legal obligation to anonymize data, there is a legal obligation to minimize the use and disclosure of personal information to what is necessary to achieve the purpose for which the data were collected. This could be interpreted to mean that if it is possible to anonymize without jeopardizing the intended purpose of processing, you must.
Let’s now turn our attention to why anonymization is especially important, yet at the same time especially hard to do in the context of AI development and use.
AI requires a lot of training data, more than the human mind can easily imagine. That makes it difficult to know what personal information is in the data set, given that, for the most part, these data are often automatically scraped from the internet, rather than cherry-picked.
Be aware that the legality of data scraping is currently a hot topic, especially in the EU, with differing views. According to Canada’s Office of the Privacy Commissioner’s Principles for responsible, trustworthy and privacy-protective generative AI technologies, personal information available on the internet is not outside of the purview of applicable privacy laws.
Thus, when training data is scraped from the internet, large commercial LLMs do contain personal data even beyond those of public figures, and, importantly, this personal information can be extracted from the model through queries by an adversary. This data can also be inadvertently exposed. For a striking example of this happening, see the case of the Korean chatbot Lee-Luda, which revealed portions of its training data gathered not from web scrapes but from user messages, including intimate conversations between partners, to its users. The reason why this is possible is due to a phenomenon called data memorization. While it is not the case that LLM store their training data physically, model training does have the effect of being able to reproduce verbatim aspects of its training data.
Research is currently underway to make LLMs “unlearn” things we don’t want them to spew out in production, but these techniques are not yet at the stage to offer a full solution to the problem.
If the use case allows it, the best way to prevent privacy issues with AI is to avoid including personal information in the training data and to prevent such data from being included in model prompts. These prompts are disclosed to the model provider and may be used to improve the model, which means the prompt content could be memorized and potentially disclosed to other users in the future.
Yet, as we remarked earlier, it will depend on the use case whether anonymization of training data is feasible from a data utility perspective – if the data is needed to make the model work or be useful, removing it is not a good option.
That means if you are developing an AI system, you need to conduct a thorough analysis regarding the necessity of each data point and the overall amount of personal data required for training. Then it is recommended to remove the unnecessary data points prior to training to comply with the data minimization principle.
Private AI’s Solution in Support of Data Anonymization
Private AI can detect, redact, or replace personal information with synthetic data, leveraging its machine learning model optimized for large amounts of unstructured data. It does so in 53 languages, various different file types, and with unparalleled accuracy. The technology aids in ensuring that only essential data is utilized for AI training and operations, helping to render personal information anonymized or de-identified, or by creating synthetic data in place of personal information.
For the use of AI systems, organizations are well advised to use Private AI’s PrivateGPT, which ensures that user prompts are sanitized – i.e., personal information is filtered out from the prompts – before it is sent to the AI system. Depending on the use case, the personal information to be excluded from the prompt can be selected on a very granular level to ensure the prompt’s utility. Before the system’s answer is sent back to the user, the personal information is automatically inserted into the output, without ever being disclosed to the model.
Note that in order to achieve full anonymization, the removal of direct and indirect identifiers may not be enough, depending on the data set. In all instances, it is advisable, and required under Québec’s Regulation respecting the anonymization of personal information, to conduct a re-identification risk assessment to confirm that the re-identification risk is below an acceptable threshold.
Conclusion
This article discusses how data anonymization and minimization are complex topics, particularly in the context of AI where emerging technologies shape the ever-changing landscape with new challenges and new proposed attempts at solutions. For help navigating these challenges, reach out to Etika Privacy. We can advise you with expert guidance on the requirements and best practices you need to be mindful of, and we can connect you with Private AI’s team to get you access to state-of-the art technology to support you with practical solutions.
October, 2nd, 2024
Authors:
Kathrin Gardhouse – VP – AI and Data Governance
Bernadette Sarazin – CEO and Chief Privacy Officer